Using Artificial Intelligence to Address Benefits Fraud
Using Artificial Intelligence to Address Benefits Fraud: Q&A with Brian Bird
Ensuring benefit payments reach those in need while safeguarding them from cybercriminals is a major challenge for state and local governments.
During the pandemic, identity fraud surged as government benefit programs expanded. A September 2023 Government Accountability Office report estimates that state unemployment insurance programs paid out up to $135 billion in fraudulent claims, though the full extent of the fraud may remain unknown.
Fortunately, advances in artificial intelligence and machine learning (AI/ML) can help agencies more efficiently identify fraud, reducing false positives and decreasing delays in benefits delivery. To learn more, we interviewed Brian Bird, VP of Tax and Revenue Analytics.
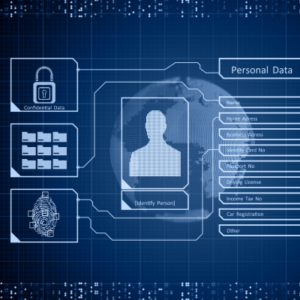
Let’s start with the basics. How can AI improve benefits fraud detection?
Unlike traditional methods that rely on static, predefined patterns drawing from historical data, AI/ML models learn and adapt over time as they are exposed to new data. AI can analyze and associate huge volumes of disparate data inputs and recognize patterns of abuse across channels in ways you simply can’t achieve manually. Graph neural networks, for example, are particularly useful in uncovering connections in organized fraud activities by identifying networks of interrelated transactions indicative of fraudulent operations.
AI/ML can also more accurately distinguish between legitimate and fraudulent transactions. Plus, using modern MLOps framework models can continuously improve and stay ahead of evolving fraudulent tactics and schemes. This improves customer experience by minimizing unnecessary transaction declines or account holds.
Unfortunately, some states have implemented automated benefit fraud detection programs that have inadvertently deprived constituents of benefits. How can agencies avoid this?
When you’re dealing with people’s benefits, you have to be careful. As one component of a multi-faceted approach to fraud detection, AI can quickly and reliably detect suspicious transactions and stop bad actors from victimizing benefits recipients. However, I would never recommend a fully automated system. At this point in its maturity, AI shouldn’t the sole arbiter of high stakes decisions, particularly determinations around benefits.
Instead of models that make automated decisions, we recommend solutions that empower and inform case workers, administrators, and benefit recipients by providing them with accurate data and insights. For example, after detecting suspicious activities, an AI solution could route cases to appropriate treatment streams, alert benefits recipients, and prompt them to use online solutions to authenticate a legitimate transaction – or confirm a fraudulent one. In a high-risk case, it could temporarily freeze their account until they can authenticate their identity with the provider.
Case workers could use detection results, risk scores, and advanced visualization tools to analyze and proactively identify vendors, employers, tax professionals, or other networks that may have been compromised by bad actors, using the data to design robust countermeasures or to alert law enforcement agencies.
Since AI-enabled fraud detection relies on datasets with sensitive attributes such as age, gender, and financial history, there’s always a potential for bias or inequity. How can agencies mitigate this risk?
Bias can creep into ML models in a variety of ways. One is through proxy data. A model that omits racial data but includes ZIP code data, for example, might still result in disparate impacts along racial lines, given the existence of residential segregation.
Another way bias can enter ML models is through training with historical data. If using data and outcomes based on human interventions in benefits delivery, any bias that might have influenced those interventions will be built into your model. In either case, the outcome could lead to disparate treatment—where decisions are applied unequally across different demographics—or disparate impacts—where decisions disproportionately harm or benefit certain demographics.
A key strategy for avoiding unfairness in AI is awareness of the risk and knowledge of data correlations that can inadvertently bias your results. The best way to keep bias out of your model is to prevent these features from entering your modeling environment in the first place. Furthermore, pre-defined strategies exist to identify measurement metrics (e.g., predictive rate parity) that can be tracked in real time to alert agencies of model drift or AI unfairness.
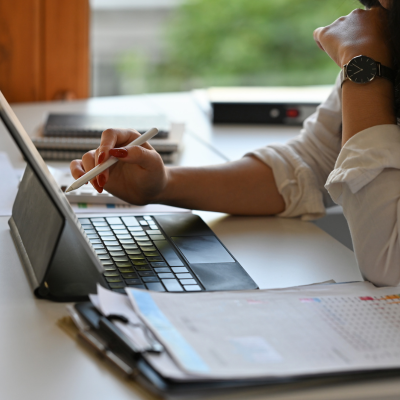
Agencies should be able to explain how certain inputs in your AI models result in certain outputs, a particular challenge in the world of modern “black box” AI technologies (e.g., Large Language Models such as ChatGPT and other deep neural networks or massive tree-based ensembles.) For instance, if a model flags an individual for identity theft, you should be able to say that 15% of the reason was because they changed their bank account, 10% was because their address change wasn’t in the National Change of Address database, 2% was because they moved to a specific ZIP code, and so on. Tools exist to estimate these impacts at the individual prediction level, and agencies will benefit from solutions that surface these insights to their humans-in-the-loop. It will give confidence to your agency and your constituents that regardless of the individual’s age, gender, and race, they weren’t flagged because of bias.
Agencies are often operating with tight budgets and resource constraints. How would you recommend they maximize early investments in benefits fraud detection?
Many states have been investing in various fraud prevention methods for years, so it’s important that any AI-powered efforts are complementary to those initiatives. As with any new program, it makes sense to prioritize problems that are painful for a wide array of participants. For example, initial AI programs could concentrate on identifying suspicious transactions or patterns within EBT card activities through ML models focused on detecting anomalous behavior. As confirmed fraud instances are uncovered, the models can be further refined and trained for greater accuracy.
Incorporating end-user complaints data into modeling early and often can also accelerate ROI. In our experience with fraud at government agencies, end-user stories and narratives about their experiences with fraud and the program in general are invaluable for understanding how fraud happens and what traces that leaves in the data. These complaints could also be connected to specific users and businesses so that case workers will have the context of the prior complaint when investigating.
-Voyatek Leadership Team