Artificial Intelligence and the Future of Government
Artificial Intelligence and the Future of Government
No technology trend has garnered more attention in the past few years than artificial intelligence (AI). While there’s been a surge in buzz around generative AI, we’ve only scratched the surface of AI’s total impact. AI encompasses a wide array of overlapping tools and architectures, including machine learning, neural networks, natural language processing, and robotic vision. The pace of AI innovation is unprecedented, potentially outpacing the velocity of Moore’s Law, which predicted that the number of transistors in an integrated circuit would double every two years.
In a government context, the question is not whether AI will impact and improve the way government services are delivered, but how and where. The challenge will be in determining what areas of AI should command valuable focus and resources, and in ensuring that government’s use of AI adds efficiency and value while preserving or enhancing the fair and equitable treatment of citizens.
Defining Artificial Intelligence
AI is the ability of applications, systems, or machines to perform tasks that typically require human intelligence. These tasks may include learning, reasoning, problem-solving, perception, language understanding, and decision-making. AI systems aim to simulate human cognitive abilities and adapt to new situations by analyzing vast amounts of data, recognizing patterns, and making predictions or decisions based on that analysis.
In the last 20 years, Voyatek’s initial predictive models have evolved into AI-based machine learning algorithms. Our solutions have helped state, local, and federal governments prevent thousands of opioid deaths, save billions of dollars in the prevention of fraud, and generate millions of additional dollars through more optimized collections and audit processes.
Using the strategy outlined in the coming pages, we’ll continue to help government harness the transformative power of AI to drive positive outcomes for citizens while ensuring safe and responsible collaboration between humans and machines.
Defining AI
Artificial Intelligence (AI) is not human intelligence. It is a collection of innovative tools that can be leveraged to accelerate and, where appropriate, automate positive outcomes for our clients and their constituents, our people, and our company. Voyatek seeks to harness this transformative power ethically, sustainably, and appropriately to create safer, healthier and more prosperous communities.
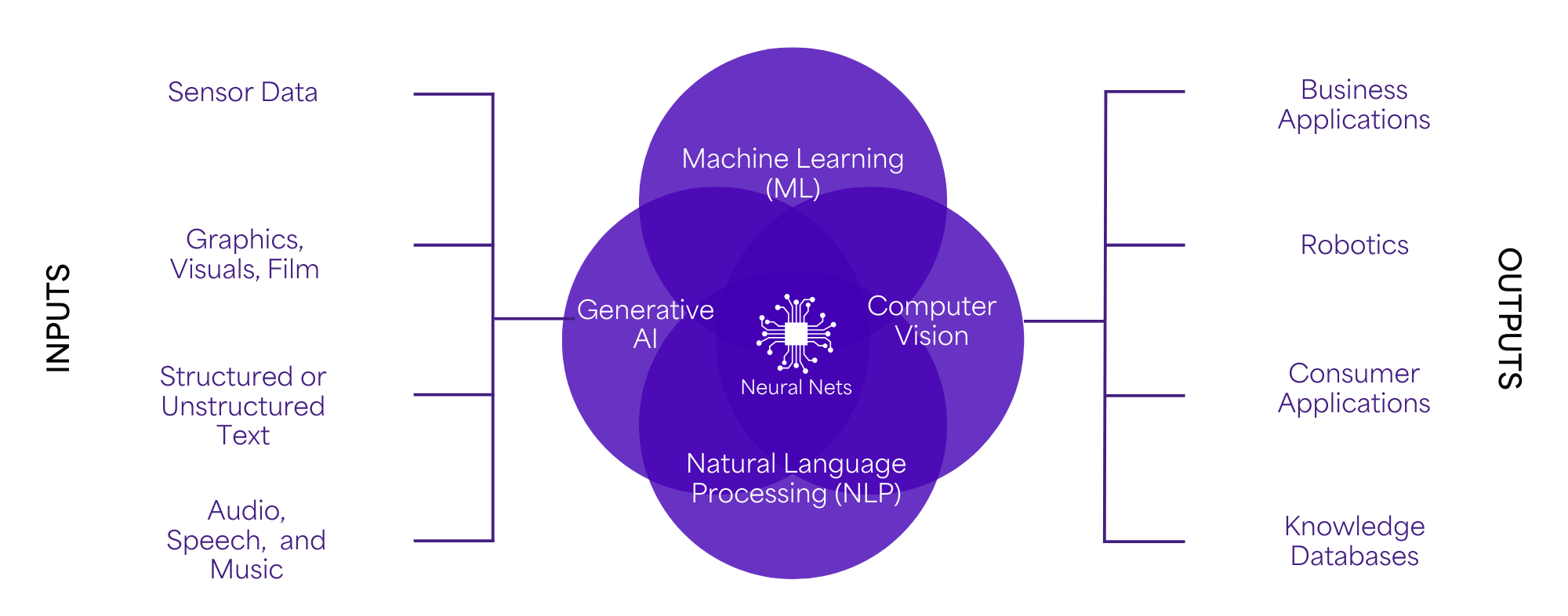
Voyatek’s AI Strategy
Voyatek’s AI strategy is built on four fundamental pillars: Outcomes First, Data Quality, Interoperability, and Risk Mitigation. By adhering to these pillars, Voyatek aims to harness AI’s transformative power while safeguarding public trust.
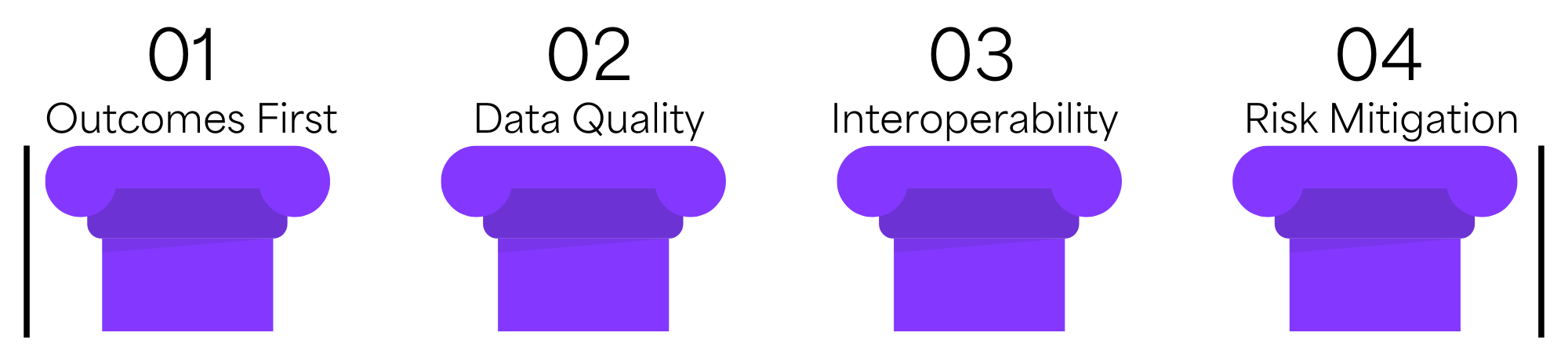
Outcomes First
When measuring the success of an AI deployment, we focus on outcomes—not outputs. While tracking transaction volume or tasks completed is essential, real-world outcomes accurately measure success. Any efficiencies or cost-savings should ultimately impact people – better access to resident services, improved community safety, and decreased employee turnover can directly affect the quality of life. Focusing on actual results ensures that AI technology is not implemented for its own sake but aligns with the government’s strategic goals and the community’s needs.
In some cases, focusing on outcomes might require working with processes and programs governments already have in place, especially considering resource, staff, and budget constraints. Toward that end, we develop our AI solutions to be modular by nature and engage closely with stakeholders at the outset to understand workflows and requirements. We use open-source, flexible software and design our solutions around widely accepted industry standards, protocols, and data formats such as JSON and XML.
Voyatek’s enterprise solution for tax and revenue agencies, RevHub, leverages AI / ML to automate the detection of non-compliance, resulting in more revenue, less fraud, and increased staff productivity:
- Increased annual collections by $800M and reduced correspondence costs by 32% for the IRS
- Prevented more than $200M in fraudulent payments and decreased individual return processing times by 93% for the Arizona Department of Revenue
- Helped to generate $480M in new revenue in California alone
Data Quality
AI does not eliminate the reality of “garbage in, garbage out.” Poor data quality (e.g. data that is incomplete, inconsistent, or biased) diminishes the performance and reliability of AI models. One of the more egregious effects are AI hallucinations, which occur when an AI system generates information or responses that are incorrect, unfounded, or nonsensical, but presented as if they were accurate. For government applications, these hallucinations could be catastrophic. A well-known recent example occurred last year when lawyers attempted to use ChatGPT for research and ended up citing past court cases that never existed. As of June 2024, data from Vectara’s Hallucination leaderboard, which uses the firm’s Hughes Hallucination Evaluation Model, shows that the typical large language model (LLM) hallucinates about 3-5% of the time.
To that end, we work with stakeholders at the beginning of each project to identify data sources that are high quality and contain the diversity required for the models. As we recognize the appropriate AI/ML algorithms to employ, we focus on choosing the suitable model complexity for the task. A simpler model is often better because it is more transparent and less prone to overfitting. Smaller models require less processing power and thus reduced costs. Further, with training, smaller models can adequately answer targeted questions comparable to large models. We work with stakeholders to create metrics for accuracy and fairness that we can frequently monitor. Finally, we regularly update and retrain models with new data to maintain and improve their accuracy over time.
Interoperability
With a solid focus on integration and interoperability, organizations can avoid creating disjointed systems that can hinder AI deployments’ accuracy and overall performance.
Integration and interoperability ensure that various systems and technologies work seamlessly together, maximizing the value derived from AI initiatives. Integration allows different software solutions and platforms to communicate effectively, facilitating the free flow of data essential for advanced AI analysis and decision-making. Interoperability, on the other hand, ensures that diverse AI systems and tools can operate together cohesively, reducing silos and increasing collaboration across departments.
We take an API-First approach when developing AI solutions, producing robust, well-documented APIs for secure and efficient communication between our solution and existing government systems. We also recognize the critical importance of security in government systems and implement strong authentication and encryption methods to maintain the integrity of interwoven systems. Finally, we incorporate continuous testing and validation throughout the integration process, including unit tests, integration tests, and end-to-end system tests. Thorough testing ensures that the integration functions as intended and that we identify and resolve issues promptly.
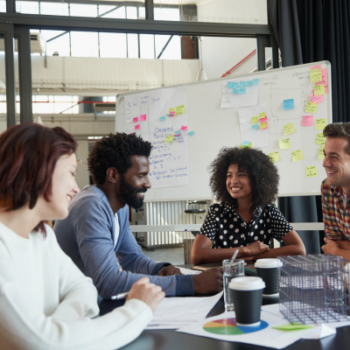
Risk Mitigation
Every AI/ML solution has the potential to produce unintended consequences. This can occur in generative AI via hallucinations or in machine learning/predictive models via false negatives or false positives. For example, a healthcare model’s incorrect diagnosis could result in unnecessary medical interventions.
While there are many concerns around AI that are typically beyond our control, e.g., environmental concerns, societal disruption, disinformation, etc., we are keenly aware of how our projects and solutions are vulnerable to privacy concerns, security vulnerabilities, and bias and discrimination.
Privacy Concerns: To minimize privacy concerns, we build the following processes into our implementation plans:
- Document and catalog the data the AI/ML has access to.
- Limit data collection to only data that is strictly necessary.
- Be transparent and accountable by communicating the purpose for the data associated with algorithms and who has access to it.
- Implementing robust security measures to protect sensitive data (PII, HIPAA, FERPA, etc.) including anonymization, encryption, access control, and regular security assessments.
- Continuously monitoring systems for new privacy risks and vulnerabilities with mechanisms in place to quickly adapt and update privacy practices as needed.
Security Vulnerabilities: In concert with privacy concerns above, we incorporate robust security frameworks (e.g., NIST 800-53) into our implementations which includes timely scanning, patching and penetration testing.
Bias and Discrimination: Using established frameworks for algorithmic bias monitoring, such as the NIST AI Risk Management Framework and GAO’s AI Accountability Framework, we take the following approach to mitigate algorithmic bias:
- Identify Fairness Metrics: We work with stakeholders to identify and answer questions that affect AI fairness such as, “what groups or sensitive attributes may be impacted?” We also catalog potential sources of bias, which can include issues like selective labels (i.e., the instances for which outcomes are observed do not represent a random sample of the population) or insufficient or inconsistent data collection.
- Monitor Fairness in AI: We use open-source tools such as AI Fairness 360 to assess, analyze, and track fairness.
- Deploy Mitigation Strategies: We implement methods to address AI fairness issues that consider trade-offs between accuracy and fairness, expected impact, and other factors.
Use Case: Avoiding Bias in Tax Fraud Detection
While AI is a technological cornerstone of closing the tax gap, agencies must beware of the possibility that bias will seep into their models. This can happen in two main ways: through proxy data (i.e., zip code data resulting in racially disparate impacts due to residential segregation) and historical data (i.e., training on a data set that already has bias). Bias is particularly difficult to avoid when agencies rely on black box algorithms, which is why visibility is so important.
Agencies should opt for models that use an open-source game-theory methodology, like Shapely Value, that clearly explains how much each data point contributes to the algorithm’s output. If a model flags an individual for tax fraud, the agency should be able to identify the contributing variables down to the percentage point.
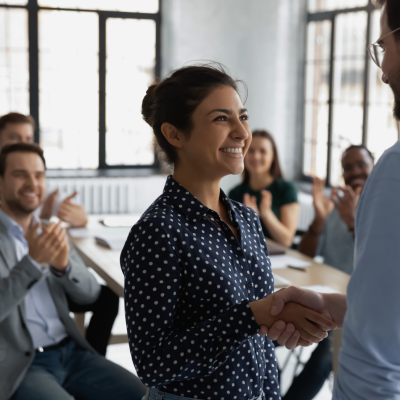
Voyatek’s Financial Aid Module, part of our Student Success Analytics solution, uses machine learning to automate identification of applications with a high potential of fraud.
When implemented by College of the Canyons, our solution correctly identified 96% of fraudulent applications preventing nearly $172,000 in fraudulent payments in a single year.
In addition to the financial savings, the college has greatly reduced the amount of time and staff dedicated to manual review and is now able to focus more resources on supporting students.
Governments Must be Proactive
Artificial Intelligence is a collection of promising new tools. The outputs from the latest generative AI applications like OpenAI’s ChatGPT, Google’s Gemini, Microsoft’s Copilot, and AWS Q are merely the tip of the iceberg. Unlike prior waves of innovation that typically required extended periods for adoption and integration, generative AI has quickly permeated various industries and highlighted what’s possible with AI more generally.
Indeed, many believe we are now living in the next Industrial Revolution, where drastic and fast advancements in technology occur rapidly, transforming the world around us. Whereas the first Industrial Revolution introduced advances in years, the AI Industrial Revolution is introducing advancements in months.
Governments need to be proactive in adapting to these changes. To achieve institutional or enterprise value, a domain-specific approach—which incorporates rather than replaces our clients’ deep institutional knowledge—is the key to achieving positive results. Furthermore, effective data management, governance, and risk mitigation are essential to applying these tools safely, responsibly, and transparently, ensuring the best outcomes for our government partners and their constituents.
-Voyatek Leadership Team